In fact, small may be beautiful if your goal is building effective solutions. We have gotten so used to the term ‘big data’ that it sometimes feels like this is the only tool available for harnessing the power of advanced data analytics and artificial intelligence. However, much smaller volumes of high quality data and well-designed infrastructure can provide the fuel for significant transformation and growth of your business.
Large companies like Google, Amazon, Netflix, Apple, and Spotify, use big data to make better decisions. Google customise ads shown to us based on our search history and geographical location. Amazon tracks what purchases we make and how much money we spend to personalise our shopping experience. Spotify analyses our listening habits to recommend us music we may like.
Are only those companies in a position to take full advantage of AI, as they already accumulate a lot of data and own a big data infrastructure? What if your company has smaller datasets, can it still utilise AI to improve its business? Absolutely. With smaller amounts of high-quality data and properly designed infrastructure, you can maximise the value of your data for your business.
If you’re working with smaller datasets then this also offers the opportunity to establish all you need to support, not just your first initiatives on implementing data-driven solutions, but all of your long-term ML/AI goals too. And it’s this firm foundation that is going to give you much more scope for success. That is why if you’re at the jumping off point for an AI project then there are some very good reasons to take time and assess the data you already have and your current infrastructure, shifting your focus from volume to quality.
There is a lot you can do with not a lot of data
AI has already had a massive impact in areas such as online advertising and web search. However, one of the major obstacles to adoption for many enterprises is the perception that, in order to build AI, you need an enormous datasets and infrastructure. CEOs and CIOs frequently get stuck here before launching an AI project, obsessing about how the business needs a year or two to build an IT infrastructure overflowing with data – and that everything should be put on hold until that point.
In fact, there is a lot you can do with only a modest amount of data. And waiting has its drawbacks. Don’t wait for the ideal conditions when it comes to AI, just make a start. The data you collect will inform the data you need to collect in a satisfying self-perpetuating cycle.
Spending two or three years to build a beautiful data infrastructure means that you’re lacking feedback from the AI team to help prioritise what IT infrastructure to build…it is often starting to do an AI project with the data you already have that enables an AI team to give you the feedback to help prioritise what additional data to collect.
– ANDREW NG, CEO and Founder at Landing AI
Reframing data – ‘big’ vs ‘good’
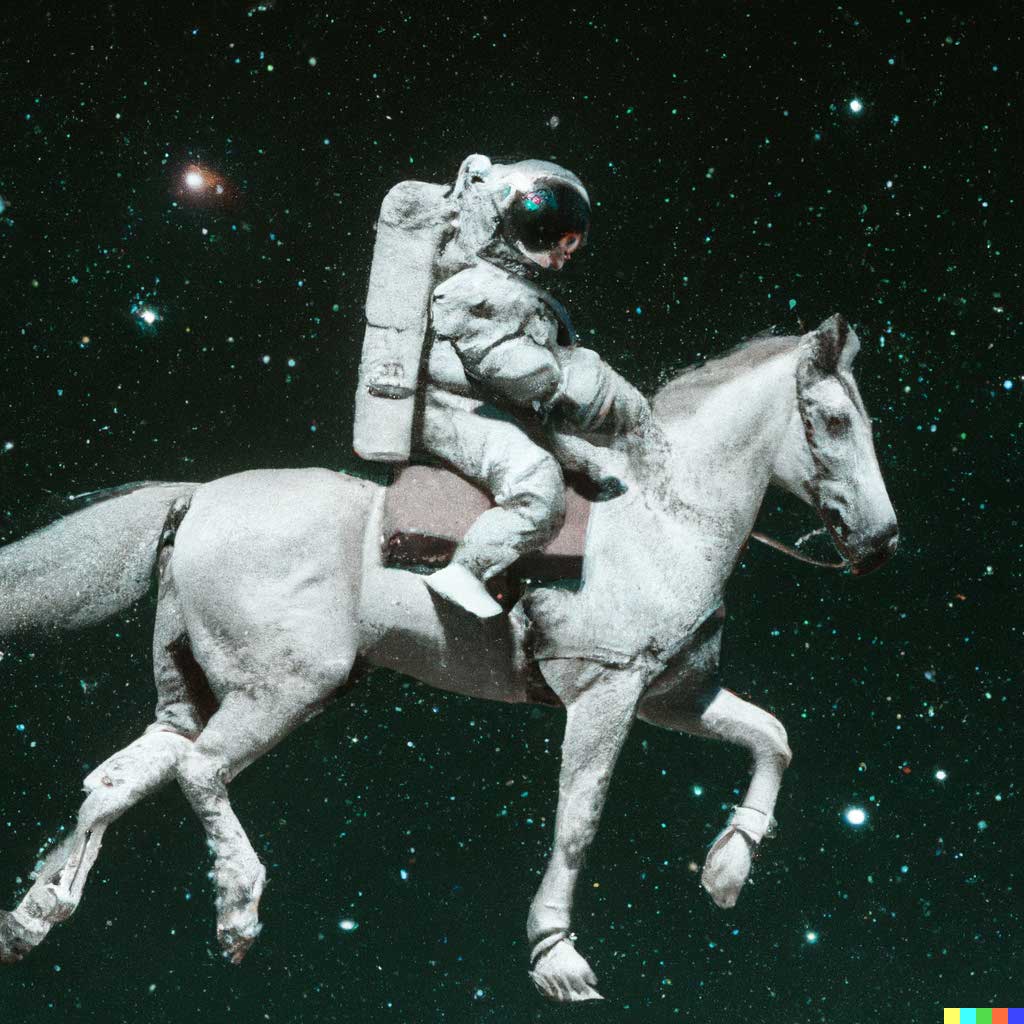
Ever-greater volumes of data have powered the most significant advances in deep learning and AI over the past decade. Thanks to the increasing size of available datasets, both computer vision and natural language processing models can constantly evolve to enable new, exciting applications. DALL·E 2, for example, is a new AI system from OpenAI that can take a simple, natural language text description and bring this to life as original, realistic images and art... Obviously, big data will always have a role to play but it has its own issues, not least the cost of data processing and the need to compute bandwidth. And some problems actually need small data solutions.
You don’t need to have access to big data to achieve innovation. In industries where it is impossible, or very expensive, to collect big datasets, AI can still make an impact, even with just a few dozen thoughtfully engineered images. We are already seeing plenty of evidence that carefully cleaned datasets with accurate labels – rather than voluminous datasets – often produce quicker improvements in model performance. That’s why focusing on data quality could enable companies with even limited data to realise the business value of AI and move data-driven projects from proof-of-concept to full scale production.
How to ensure the highest quality data – and why that's important?
This question turns on the specifics of your business – and the challenges that you would like to use data to address. However, in our experience, there are steps any organisation can take to improve the potential for developing successful data-driven projects:
- Analyse how you collect data. Even a minor improvement in data collection could be the difference between successful and failed projects. Cleaning your microscope camera before acquiring the images could help you avoid time on developing complicated denoising algorithms. Changing illumination conditions could help you improve the performance of your segmentation algorithms.
- Ensure a reliable data flow. By thoughtfully designing data streams and ETL processes, you can reduce the number of errors in data and improve the overall efficiency of your team. How much effort does it take for your data science team to get access to data? If your analysts rely on some manual Excel exports generated by another teams, there is likely a lot of opportunities for improvement in your organisation.
- Examine your data. Understanding the data you have could help you identify why an AI system could fail further down the line and help you come up with the right approach to avoid the problem. For example, the failures of a classifier on one specific class may indicate that you need to provide more annotations for this class or redefine this class potentially splitting it into several subclasses and retrain the model.
What's next? Doing more with your data
Engineering the best datasets, training a model, and deploying your AI solution in production might sound like a complete process. However, it’s just the start of the journey. The world is dynamic, environments change and upgrades and evolution are constant – and your data driven infrastructure needs to reflect this.
For example, lighting systems in a production environment may be replaced, resulting in the visual system controlling product quality triggering more false positive alerts. Or users might start using new words not yet known by your NLP system, resulting in misleading answers that make them rethink their decision to use your services.
Your system should be able to detect any changes in input data, trigger alerts, and automatically indicate that the model needs an update. That is why building a data driven solution isn't just a one time investment. It is, necessarily, an ongoing process that will thrive or fail depending on whether you have the right support – and, of course, how much of a priority good data is to you.
At Samuylov.ai we are ready to support your business navigating through each step towards advanced data analytics. We take an individual approach to find a right balance between quantity vs quality of the data you need to bring your ideas to life. We will work with you to create the team, infrastructure and technology that you need to achieve your ML/AI goals.